Agentic AI has growing potential in Upstream
In conversations with the “techier” side of oil and gas, excitement over large language models - like chatGPT - is giving way to the prospects of “agentic AI”. Agentic AI differs from traditional large language models like ChatGPT in its ability to act autonomously on specific tasks, rather than simply processing and generating responses to prompts. While models like ChatGPT rely on user inputs to guide their functionality, agentic AI integrates specialized "agents" that are trained for particular operations, such as seismic analysis or predictive maintenance.
These agents can interpret, process information, and take action, often connecting with operational tools and workflows to execute tasks in real-time. This task-specific design allows agentic AI to perform complex, multi-step processes independently, making it a more dynamic and actionable tool than current LLMs.
- Perception: AI agents gather and process data from various sources, such as sensors, databases, and digital interfaces. This involves extracting meaningful features, recognizing objects, or identifying relevant entities in the environment.
- Reasoning: A large language model acts as the orchestrator, or reasoning engine, that understands tasks, generates solutions, and coordinates specialized models for specific functions like content creation, vision processing, or recommendation systems. This step uses techniques like retrieval-augmented generation (RAG) to access proprietary data sources and deliver accurate, relevant outputs.
- Action: By integrating with external tools and software via application programming interfaces, agentic AI can quickly execute tasks based on the plans it has formulated. Guardrails can be built into AI agents to help ensure they execute tasks correctly.
- Learning: Agentic AI continuously improves through a feedback loop, or "data flywheel," where the data generated from its interactions is fed into the system to enhance models. This ability to adapt and become more effective over time offers businesses a powerful tool for driving better decision-making and operational efficiency.
Think of agentic AI as a team of highly skilled specialists versus a general advisor. A large language model like ChatGPT is akin to a consultant who can answer a wide range of questions, provide insights, and guide decision-making based on the information you provide. However, it depends on you to decide how to act on that advice.
In contrast, agentic AI is like having a team of specialized experts—each trained to handle a specific task, such as analyzing seismic data, predicting equipment maintenance needs, or optimizing workflows. These "specialists" not only provide recommendations but also act autonomously, executing tasks in real-time and adjusting their approach based on new data or circumstances. While the consultant gives you the blueprint, the specialist team builds the solution for you. This autonomy and task-specific expertise make agentic AI more proactive and operationally integrated.
In upstream, agentic AI offers immense potential to revolutionize exploration, production, and sustainability. By analyzing massive datasets at unprecedented speeds, AI agents can streamline operations such as seismic analysis, reservoir modeling, and real-time monitoring. These technologies reduce reliance on manual processes, lower costs, and enhance operational precision, contributing to safer and more sustainable practices in an industry under increasing environmental scrutiny. Early adopters are already seeing results in the field. With the United Arab Emirates ADNOC's ENERGYai initiative has demonstrated 20-30% improvements in drilling efficiency and 15-25% reductions in maintenance costs.
In land and data management specifically, agentic AI excels in solving historically complex challenges. Seismic data analysis, a cornerstone of exploration, can now be performed in hours instead of months, significantly accelerating project timelines. AI-driven tools also enhance reservoir characterization by integrating diverse datasets into cohesive models, improving predictions of hydrocarbon recovery and resource utilization. Furthermore, AI agents are also being used to manage land lease agreements, monitor regulatory compliance, and identify risks in land use, making the administration of large-scale operations more efficient.
While the applications are exciting and generate deeper and more valuable automation potential, their complexity and required data infrastructure is well beyond other forms of large language models. Such complexity means that adoption will be spotty, but agentic’s impact - and the amount you hear about it - are likely to rise through 2025.
Related News & Articles
View All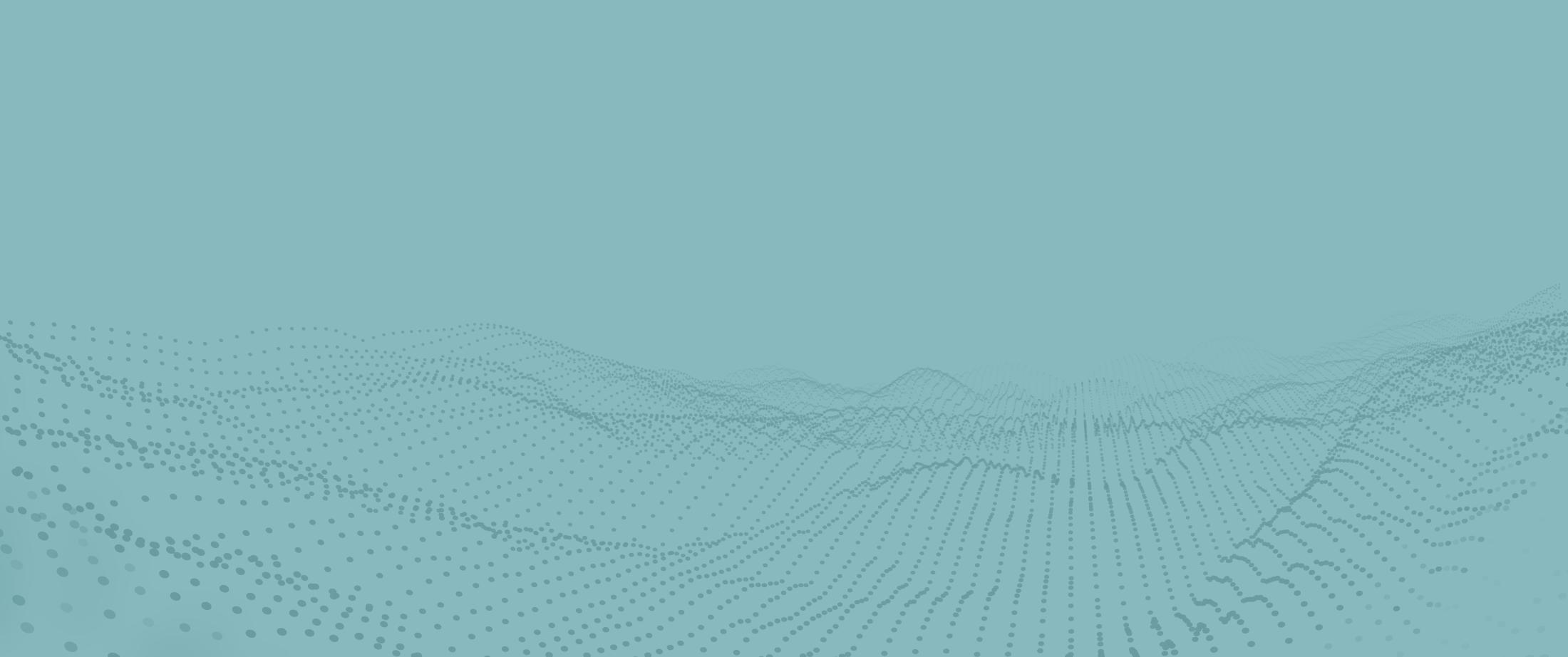
Subscribe to go deep
Upstream Intelligence is our weekly overview designed to keep you up to date on the most interesting and important trends impacting U.S. energy producers. Sign up today for your subscription!